AI-Driven Data Extraction: Revolutionizing Medical Research
Artificio
Automation
May 13th, 2024
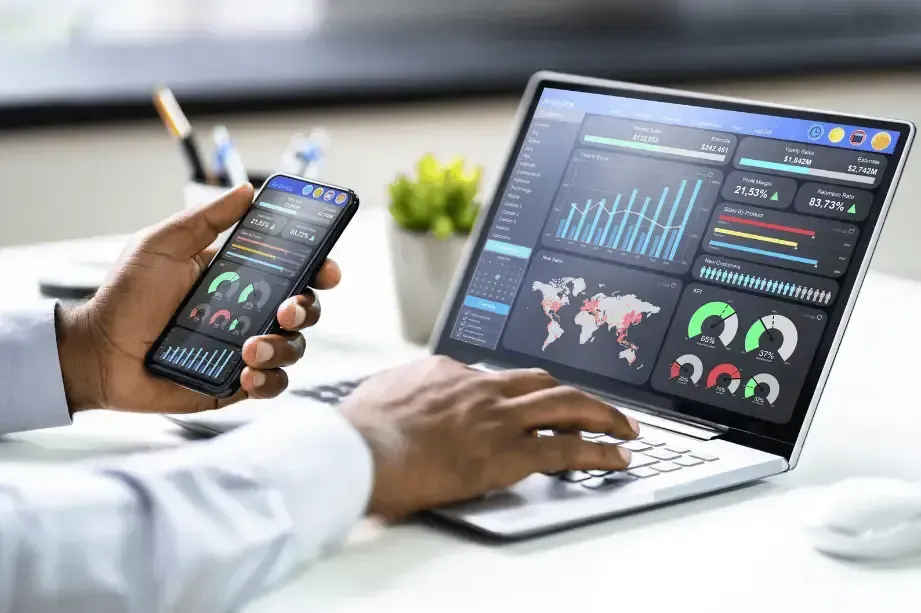
Share:
Category
Explore Our Latest Insights and Articles
Stay updated with the latest trends, tips, and news! Head over to our blog page to discover in-depth articles, expert advice, and inspiring stories. Whether you're looking for industry insights or practical how-tos, our blog has something for everyone.